The Impact of Zero-shot Recommendations
9 December 2024 | 5 min
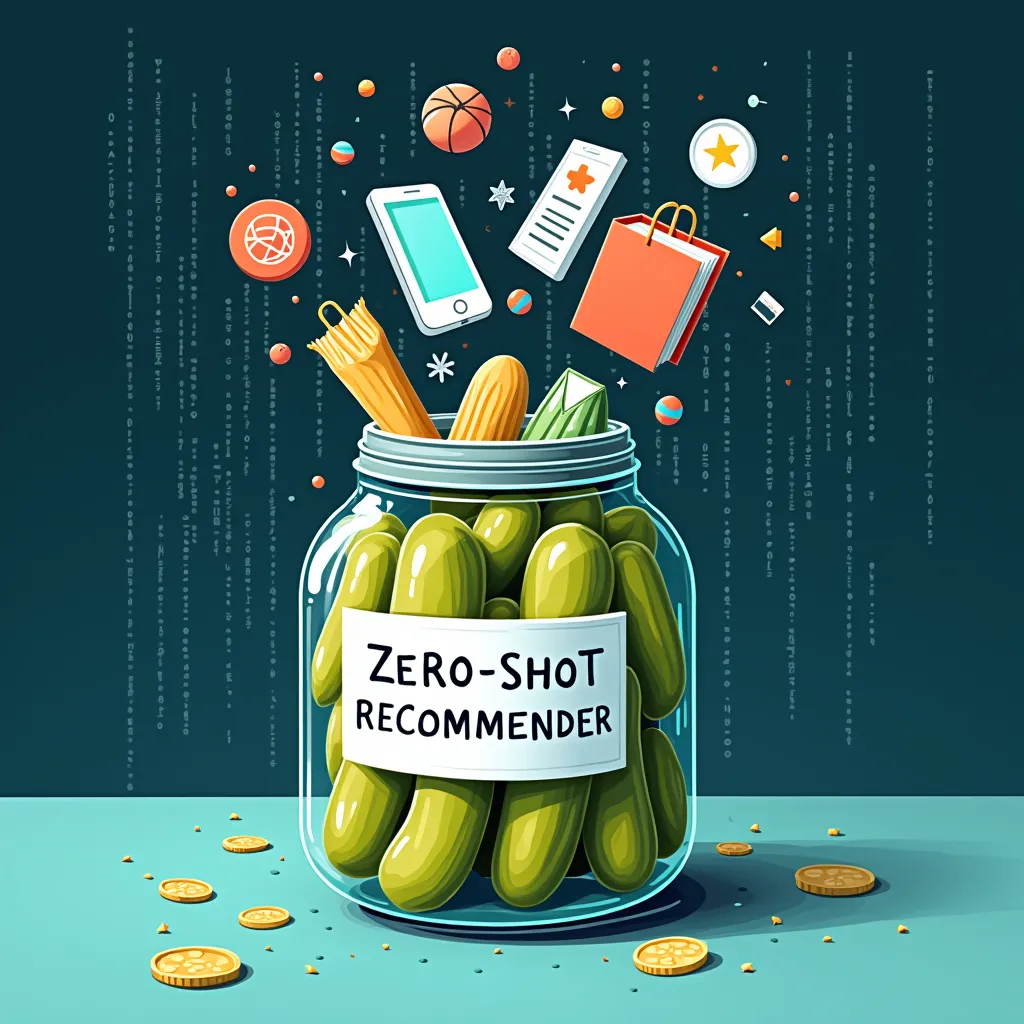
The trajectory of recommender systems mirrors the broader evolution of AI: a journey from narrow, data-hungry models to increasingly flexible and efficient architectures. As we enter a new era of AI-powered discovery, zero-shot recommendations emerge as a step-change in how businesses connect users with relevant content and products- reducing fatigue and improving conversion rates in large, evolving catalogues.
1. The Role of Traditional Recommender Systems
In today's digital age, recommender systems have become the invisible guides shaping our online experiences. From e-commerce giants suggesting our next purchase to streaming platforms curating our entertainment, these AI-driven systems play a crucial role in connecting users with relevant content and products.
However, traditional recommender systems, while powerful, have long grappled with significant limitations. They struggle with new users or items, often failing to provide meaningful recommendations in these "cold start" scenarios. Moreover, their reliance on historical data can lead to filter bubbles, limiting user discovery and hindering business growth. Zero-shot recommendations are a revolutionary new approach that promises to overcome these challenges and usher in a new era of AI-powered discovery.
By leveraging advanced language and vision models, zero-shot systems can make intelligent suggestions even in entirely novel scenarios, potentially transforming how businesses engage with their users and manage their ever-evolving catalogs.
2. The Dawn of Zero-shot Recommendations
Traditional recommender systems, emblematic of the AI 1.0 and 2.0 eras, rely heavily on historical user-item interactions. They excel at making predictions within known domains but falter when faced with new users, items, or contexts - the infamous "cold start" problem. Zero-shot recommendations herald a paradigm shift, enabling systems to make meaningful suggestions without prior interaction data.
The key innovation comes from our ability to use vision-language models to extract and reason over semantic information. Instead of relying solely on historical user-item interactions, these systems leverage rich, multimodal representations of items (such as text descriptions, images, or videos) to understand their characteristics and relationships. This allows the recommender to make meaningful suggestions even for entirely new items or users, based on semantic similarity and learned patterns rather than direct interaction history. By doing so, zero-shot recommenders mark a transition from narrow, interaction-based models to more flexible, content-aware recommendation engines that can generalize across domains.
As researchers tackle the challenges of zero-shot recommendations, several promising approaches have emerged.
3. The Three Paths of Driving Zero-shot Recommendation Research
Zero-shot recommendation research is advancing along three main paths:
3.1. Content-based Approaches
Researchers are tapping into rich item
descriptions and metadata to create "universal embeddings" that transcend domain
boundaries. Models like ZESREC utilize natural language
processing techniques to generate transferable item representations.
3.2. Large Language Model Integration
The vast knowledge encoded in LLMs
is being harnessed for zero-shot recommendation tasks. Models such as
P5 (Preference, Person, Product, Place, and Promotion) demonstrate how fine-tuned LLMs can generate personalized recommendations without extensive interaction
data, but rather by leveraging pre-trained knowledge and various context signals that span both
structured and unstructured data.
3.3. Hybrid Architectures
Innovative combinations of techniques are
pushing the boundaries of zero-shot capabilities.
ContrastVAE
and
TIGER, for instance, melds contrastive learning with variational autoencoders and VQAE models to
create more robust and generalizable recommendations domains.
These paths represent distinct but complementary approaches to zero-shot recommendations, each offering unique advantages and challenges, which will shape the future of AI-powered discovery in e-commerce, and beyond.
4. Overcoming the Economic Hurdles of Traditional Recommenders
The reason why zero-shot recommendations are so exciting is that they address fundamental business challenges that have long plagued traditional recommender systems:
Cold Start Inefficiency: The inability of traditional recommender systems to provide accurate recommendations for new users or items, due to the lack of interaction data, leads to low returns to user acquisition and slowing inventory turnover as you scale.
Data Sparsity: Even for existing users and items, high user churn or catalog turnover after large sales and peak shopping holidays results in a significant performance degradation for traditional recommenders, affecting the quality of recommendations and user experience for existing customers.
Scalability Bottlenecks: As user and item catalogs grow, many traditional recommender systems struggle to scale efficiently in terms of both computational resources and recommendation quality. Interactions data grows quadratically with the number of users and items, leading to increased computational costs and slower recommendation times as time goes on. This is hard to asses in advance, and can lead to significant costs and performance issues as the system scales.
Diversity Deficit: Recommender systems often fall into "filter bubbles," failing to introduce users to potentially interesting new content. This can lead to reduced user engagement and lower customer lifetime value. This can harm new brand and product launches and entrench the business in existing product categories and supply relationships.
Cross-Domain Rigidity: Highly specialized recommender systems struggle to adapt to new or related product areas, limiting business expansion opportunities and cross-selling potential. This can lead to missed revenue opportunities and close off new markets for growth.
5. The Economic Impact of Zero-shot Recommendations
Zero-shot recommendation technologies have the potential to unlock new business value across industries:
Cold Start Revolution: By leveraging transferable knowledge, zero-shot recommenders can provide meaningful suggestions from day one, dramatically improving user satisfaction and engagement for new products or users, reducing the cost of user acquisition and increasing inventory turnover.
Data Efficiency: Zero-shot approaches can potentially reduce the amount of data needed to build effective recommender systems, lowering costs and time-to-market for new features.
Cross-selling Catalyst: The ability to make recommendations across domains could significantly boost cross-selling opportunities, increasing customer lifetime value, and unlocking new streams for growth.
Market Expansion Accelerator: Companies can more easily expand into new product categories or markets, as zero-shot recommenders leverage existing knowledge to bootstrap recommendations in unfamiliar domains.
Long-tail Discovery: Zero-shot methods may excel at surfacing niche or long-tail items that traditional systems struggle with, potentially increasing inventory turnover and user satisfaction.
Hyper-personalization at Scale: As zero-shot recommenders mature, they could enable truly personalized experiences for every user, even in scenarios with limited data.
Privacy-Centric Innovation: By relying less on individual user data and more on transferable patterns, zero-shot recommenders may be better positioned to thrive in an increasingly privacy-conscious regulatory landscape.
The dawn of zero-shot recommendations marks a pivotal moment in the evolution of AI-powered discovery. By addressing the fundamental limitations of traditional recommender systems and offering unprecedented flexibility, these technologies promise to drive significant business value across diverse industries. As research in this field accelerates, we can expect to see increasingly sophisticated and effective zero-shot approaches reshaping the landscape of personalized recommendations.